Abschlussarbeit
Exploring generalization of deep neural networks for the semantic segmentation of burnt areas across Sentinel-2 and Sentinel-3 satellite imagery
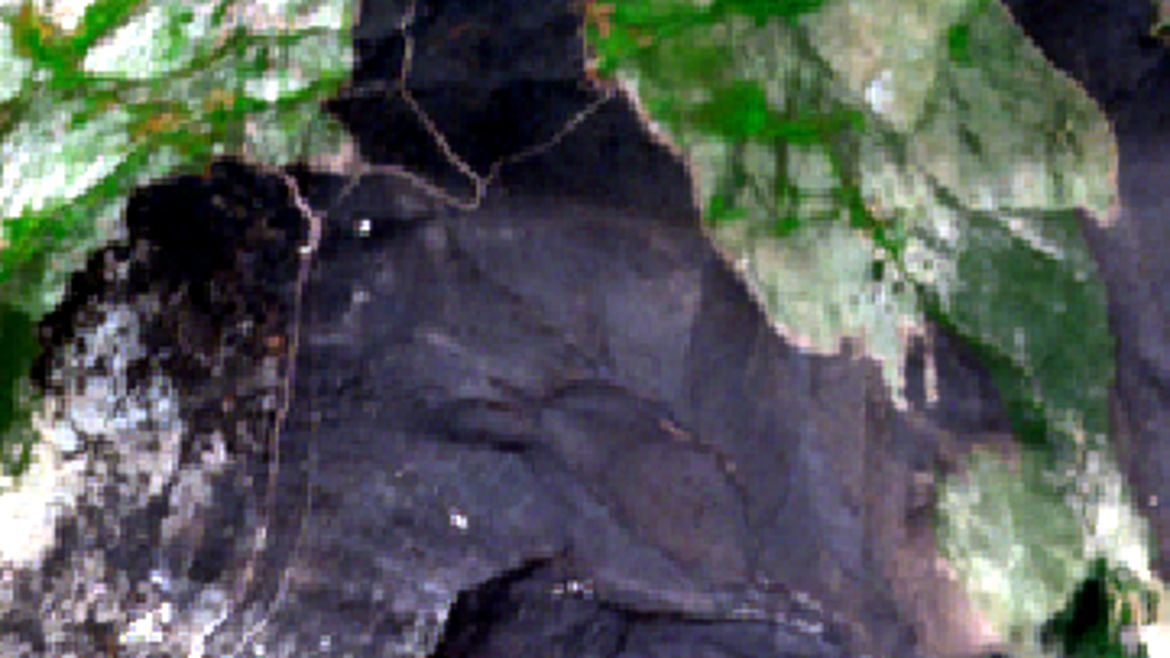
Can we train a convolutional neural network so that it automatically detects recent wildfires in multi-sensor satellite images? Are the results comparable to zero-shot AI methods like the Segment Anything Model?
Details
- Extern/e Autor:in
- Simon Worbis
- Extern/e Betreuer:in
- Dr. Michael Nolde, Dr. Marc Wieland (DLR)
- Intern/e Betreuer:in
- Prof. Dr.-Ing. Andreas Schmitt
- Abschluss
- Master
- Studiengang
- Geomatik
- Jahr
- 2024
- Fakultät
- Fakultät für Geoinformation
- Status
- abgeschlossen
- Themengruppe
- Photogrammetrie_Fernerkundung
- Weiteres
-
#extern
Low-resolution data can complement resources for mapping burnt areas and provide higher temporal coverage in Earth observation, which is very important for rapid emergency response. This thesis focuses on examining model transferability across Sentinel-2 (MSI) and Sentinel-3 (OLCI) related data for the semantic segmentation of burnt areas in order to gain a better understanding of how generalization behaves across similar optical sensors and what affects performance. Several approaches including data augmentation, fine tuning and joint learning are investigated. In addition, there is a need to evaluate the capabilities of existing foundation models for their applicability in the field of remote sensing to determine whether they are already suitable for segmentation in this area.
In this respect, the models developed are compared with the Segment Anything Model (SAM).
Sentinel-2 (S2) and Sentinel-3 (S3) image domains differ especially in their spatial resolution due to a different Ground Sampling Distance (10 m vs 300 m) of the MSI and OLCI sensor (figure 1), which is considered the main challenge of the task at hand. Filtering the satellite images of both domains to the blue, green, red and near-infrared channels, which are used jointly by the MSI and OLCI sensors, enables different experiments and a direct comparison of applied approaches.
Comparisons of the approaches show that a model’s generalization with respect to a different sensor can be increased. By means of the comparable setup and two baseline models, which were trained on Sentinel-2 and Sentinel-3 data, respectively, it is determined that the methods are useful in different ways. Data augmentation and joint learning can reduce the domain shift with respect to Sentinel-3 data (target domain) between 16 to 25 percent, while maintaining accuracy on Sentinel-2 data (source domain) compared to the baselines. Fine tuning is also suitable for generalizing to the target domain, but at the expense of accuracy to the source domain. Overall, a higher ability for a model to generalize well can be observed when using a combination of data augmentation and joint learning. The results obtained by the Segment Anything Model, on the other hand, are significantly less accurate.
Cooperation with: Germn Aerospace Center (DLR), Earth Observation Center (EOC), Oberpfaffenhofen
Supervisors at DLR: Dr. Michael Nolde and Dr. Marc Wieland
Abbildungen
ab-ext