Abschlussarbeit
Evaluating AI-Based Approaches for Doline Detection
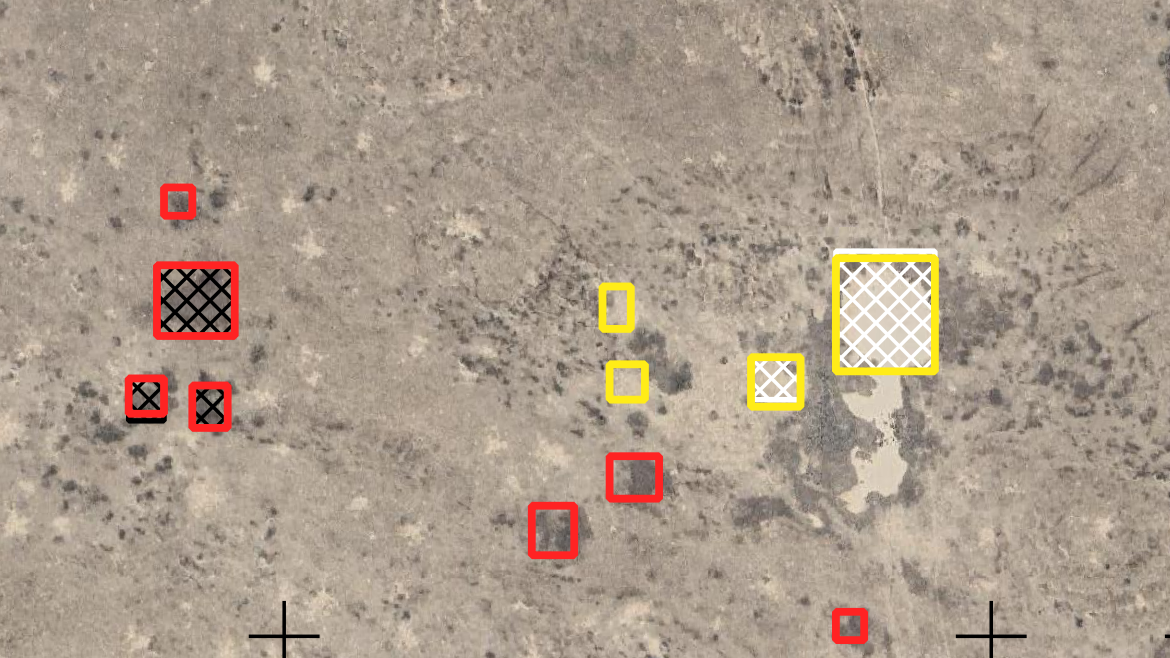
Can Artificial Intelligence help to detect dolines? How can we bring together satellite images and AI?
Details
- Extern/e Autor:in
- Marina Rug
- Intern/e Betreuer:in
- Prof. Dr.-Ing. Andreas Schmitt
- Abschluss
- Bachelor
- Studiengang
- Angewandte Geodäsie und Geoinformatik
- Jahr
- 2024
- Fakultät
- Fakultät für Geoinformation
- Status
- abgeschlossen
- Themengruppe
- Photogrammetrie_Fernerkundung
Dolines are naturally occurring funnel-shaped depressions in karst landscapes posing significant geohazard risks to human settlements and infrastructural developments. Therefore, in this thesis we present an evaluation of AI-based approaches for the detection of dolines and takirs (remarkably bright areas) in satellite imagery, focusing on the Mangystau Steppe in Kazakhstan, see Figure 1.
Specifically, we analyse the potential of four different AI models. On the one hand, we fine-tune Yolo and Faster R-CNN, two state-of-the-art computer vision algorithms designed for detecting objects by predicting bounding boxes. On the other hand we explore the capabilities of Large Language Models, concretely ChatGPT, for doline detection as well as SamGeo, a segmentation based tool. The basis for this analysis is the preparation of a high quality dataset composed of pre-processed satellite images.
The results show that, given our dataset and computational resources, the YOLO algorithm demonstrates superior efficiency and performance in doline detection (see Figure 2) in contrast to Faster R-CNN. In particular, with Yolo 74% of dolines and 86% of takirs could be detected while maintaining a high precision of 94% and 97%, respectively. Surprisingly, ChatGPT being a model designed for language tasks, shows enhanced capabilities in detecting dolines on images. On a reduced test dataset, the best prompting configuration classified all dolines and takirs correctly. SamGeo, despite being designed for geospatial segmentation tasks, performed poorly in our setup. This could be attributed to a potential mismatch between the pre-processing of our data and the dataset used for pre-training SamGeo.
Overall, this thesis demonstrates significant capabilities of AI in enhancing doline detection from satellite imagery, affirming that in remote karst regions distant from urban centers, AI-based methodologies offer a promising and efficient approach for remote sensing applications.